A comprehensive understanding of various physical and environmental processes of the urban environment, including not only the urban heat island effect and air pollution episodes but also the interactions between cities and surrounding areas, demands long-term, system-based data analytics and modeling frameworks that move beyond single-event or single-city diagnoses.
Our group examines the dynamics of urban environments and their interactions with regional-scale processes based on temporal and geospatial data analyses, machine learning methods, complex system theory, and network models driven by multiscale and multilevel data products. These include but are not limited to ground-based observational data, remote sensing products, reanalysis products, and climate model outputs.
Geospatial Data Analysis
We have applied temporal and geospatial data analytics to examine various processes within the urban environment. One example is the cooling capacity of urban trees in response to thermal extremes in major U.S. metropolitans (Wang et al., 2019). To quantify the cooling capacity, we proposed a generic cooling rate that is defined as the change in temperature per fractional urban tree cover (FTC), and for remotely sensed land surface temperature, it becomes surface cooling rate.
Based on extensive remotely sensed data during extreme heat waves and cold waves in 11 U.S. metropolitans, we found that the surface cooling rate is dominated by plant transpiration up to 1.336 °C per percentage of FTC in heat waves, and its temperature dependence remarkably resembles the thermodynamic liquid-water-vapor equilibrium. Urban trees also exert a pseudo cooling effect in cold waves in contrast to anthropogenic heat emission. The enhanced cooling capacity of urban trees under higher temperatures provides insight into this nature-based solution for better ecosystem services under projected future increases in extreme heat.
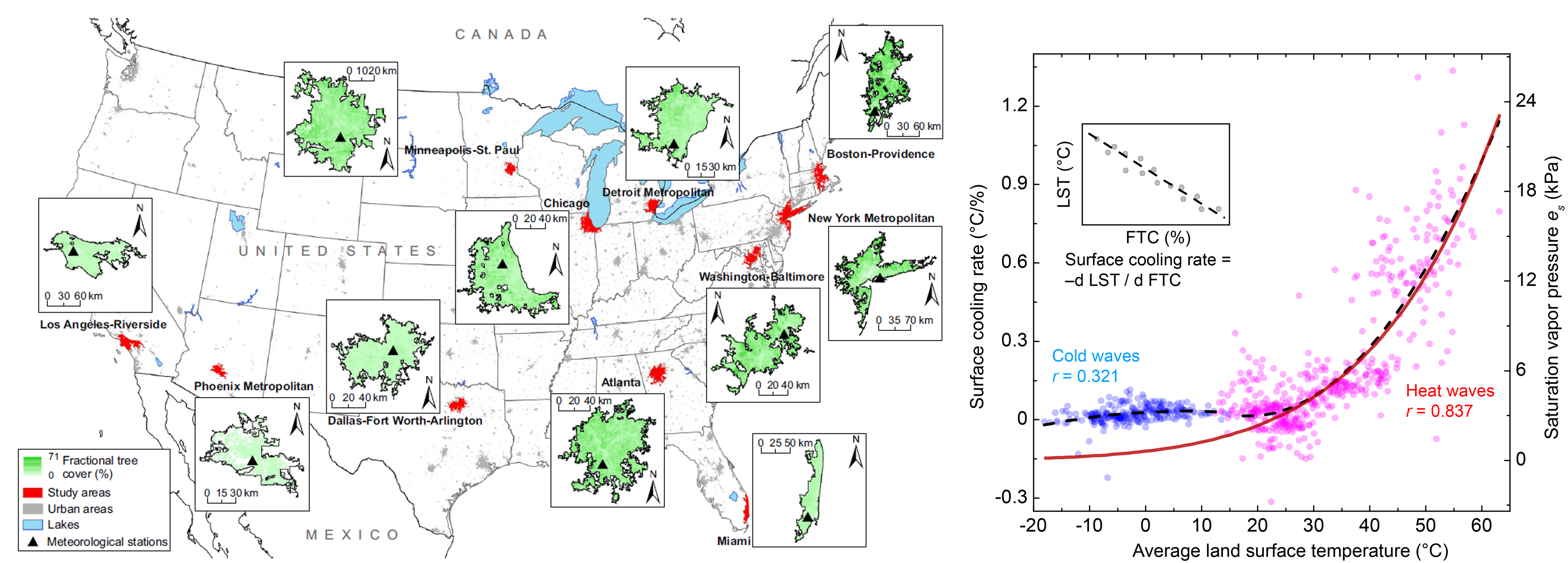
Complex Systems and Network Models
Numerous urban meteorological and climatological studies have been committed to developing and assessing mitigation and adaptation measures in response to the deteriorated urban environment. However, the effectiveness of these strategies often exhibits strong variability among cities as induced mainly by different geographical conditions.
On the other hand, urban systems are also inherently similar. For example, cities are often statistically self-similar in morphology and hierarchically organized. Cities located nearby may also share similar intrinsic stressors (e.g., societal, political, and economic changes) and external forcings (e.g., climate change). This similarity suggests potential analogous responses of cities and their components to both short-term and long-term environmental stressors, especially if their geographical or climatic conditions are alike.
Our group has leveraged complex systems and network theories to examine the response of urban environments and urban systems under changing conditions. By viewing cities and regions as highly connected or teleconnected systems, organized clusters, and/or complex networks at multiple scales, we aim to provide a more holistic, system-based view to facilitate our understanding of urban systems and potential pathways toward a more sustainable and resilient future.
The figure below is from one of our earliest system-based studies, in which we proposed a modeling framework to capture spatial similarity and connectivity among cities in response to short-term and long-term environmental stressors (extreme heat, air pollution, and precipitation) (Wang et al., 2020a). As an illustration, we used a generic message-passing-based algorithm.
We found that U.S. cities are clustered as locally and regionally connected groups, while the hub–periphery organization manifest via environmental similarity and atmospheric transport under both event-scale meteorological extremes and long-term environmental stressors.
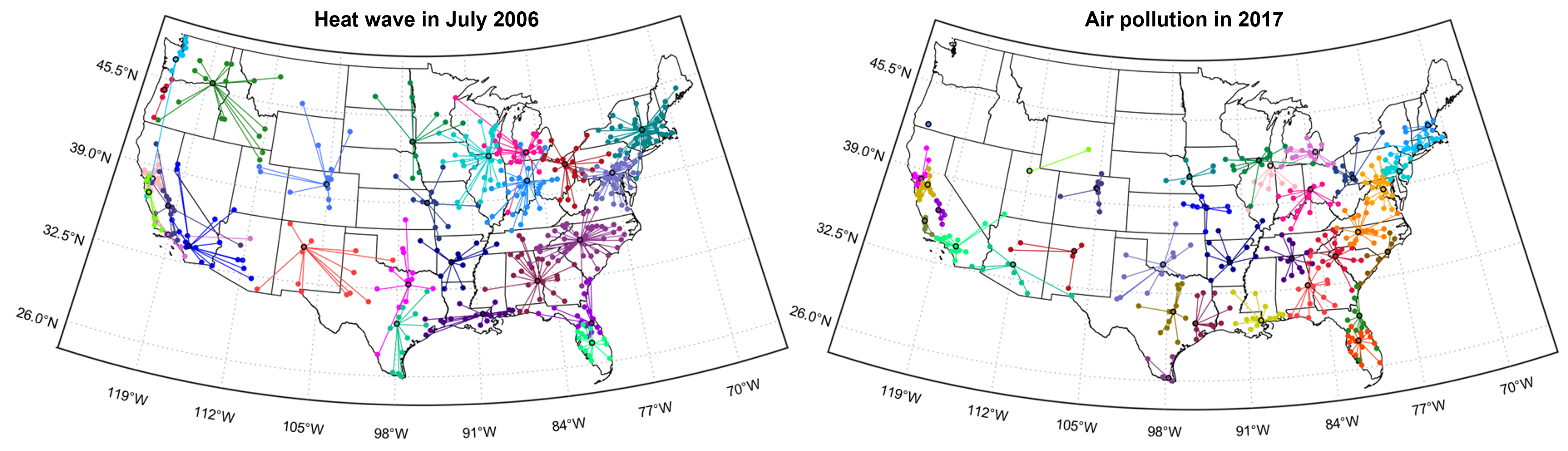
Moving from isolated clusters to connected networks, we have recently constructed climate networks for cities in the contiguous U.S. based on temperature and precipitation data to analyze their topological structure and their evolution over time. Our analyses cover not only basic topological properties (e.g., Wang and Wang, 2020), but also synchronization (e.g., Wang et al., 2021), critical slowing down (e.g., Wang et al., 2020b; Yang et al., 2022a), and causal influence (e.g., Yang et al., 2022b).
As an example, the figure below shows the urban climate networks constructed using correlations of long-term air temperature time series with a wide range of coefficient thresholds (Wang et al., 2021). We found that the urban climate networks of U.S. cities are highly modular with spatial hierarchical organization, and the small-world effect is also manifest in these networks with the characteristic path length between any pair of nodes ≤ 5.
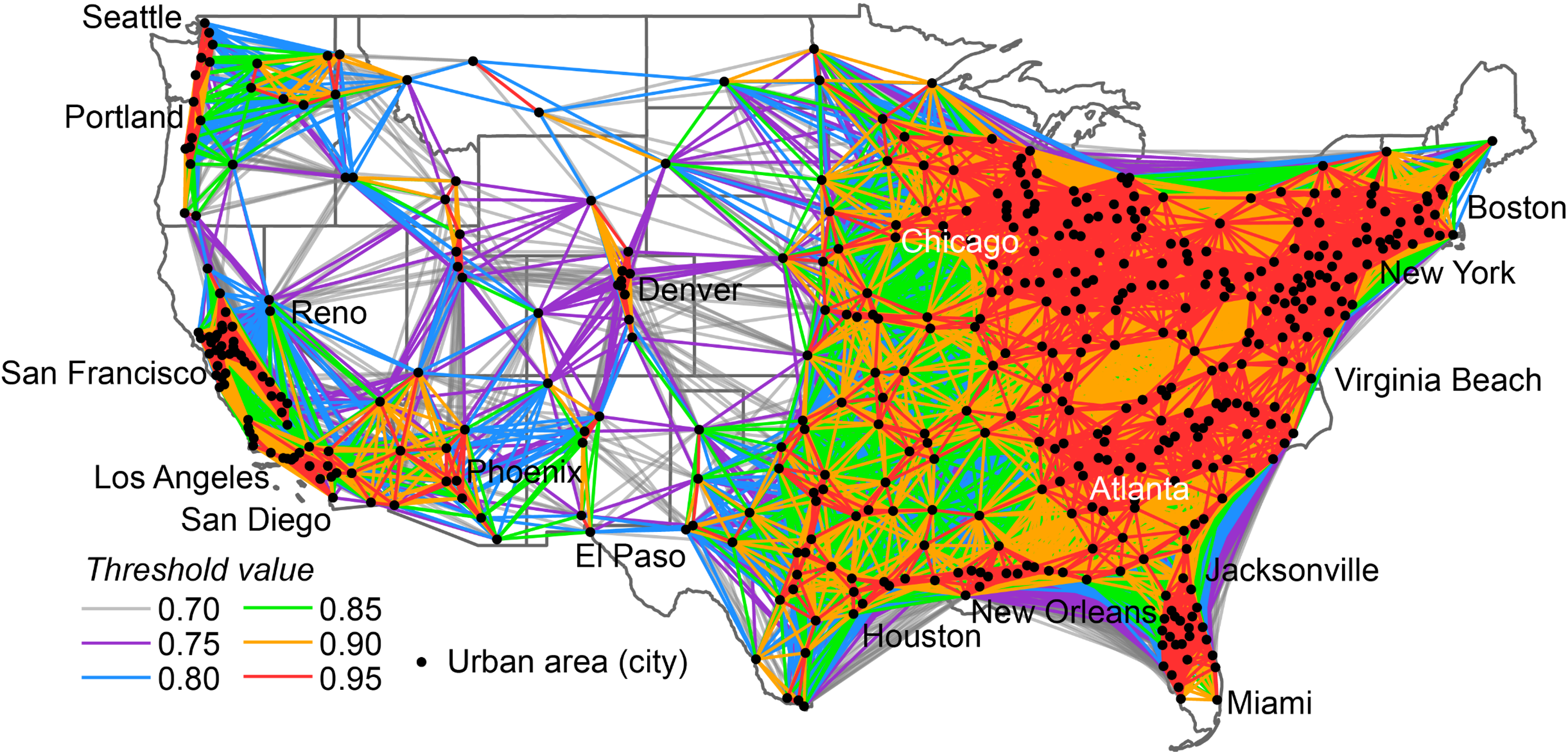
Relevant publications:
- Cordero, R. R., Feron, S., Damiani, A., Carrasco, J., Karas, C., Wang, C., Kraamwinkel, C., & Beaulieu, A. (2024). Extreme fire weather in Chile driven by climate change and El Niño–Southern Oscillation (ENSO). Scientific Reports, 14, 1974.
- Lu, M., Zhou, C., Wang, C., Jackson, R. B., & Kempes, C. P. (2024). Worldwide scaling of waste generation in urban systems. Nature Cities, 1, 126–135.
- Feron, S., Cordero, R. R., Damiani, A., Oyola, P., Ansari, T., Pedemonte, J. C., Wang, C., Ouyang, Z., & Gallo, V. (2023). Compound climate-pollution extremes in Santiago de Chile. Scientific Reports, 13, 6726.
- Yang, X., Wang, Z.-H.*, Wang, C., & Lai, Y.-C. (2023). Finding causal gateways of precipitation over the contiguous United States. Geophysical Research Letters, 50(4), e2022GL101942.
- Yang, S., Liu, J.*, Wang, C., Zhang, T., Dong, X., & Liu, Y. (2022). Vegetation dynamics influenced by climate change and human activities in the Hanjiang River Basin, central China. Ecological Indicators, 145, 109586.
- Yang, X., Wang, Z.-H., Wang, C., & Lai, Y.-C. (2022). Detecting the causal influence of thermal environments among climate regions in the United States. Journal of Environmental Management, 322, 116001.
- Ouyang, Z., Sciusco, P., Tong, J., Feron, S., Lei, C., Li, F., John, R., Fan, P., Li, X., Williams, C., Chen, G., Wang, C., & Chen, J. (2022). Albedo changes caused by future urbanization contribute to global warming. Nature Communications, 13, 3800.
- Yang, X., Wang, Z.-H., & Wang, C. (2022). Critical transitions in the hydrological system: early-warning signals and network analysis. Hydrology and Earth System Sciences, 26(7), 1845–1856.
- Li, Y., Wang, C., & Su, F. (2021). Evaluation of CMIP6 models over two Third Pole subregions with contrasting circulation systems. Journal of Climate, 34(22), 9133–9152.
- Wang, Z.-H., Wang, C., & Yang, X. (2021). Dynamic synchronization of extreme heat in complex climate networks in the contiguous United States. Urban Climate, 38, 100909.
- Zhang, F.†, Wang, C.†, & Wang, Z.-H. (2020). Responses of natural vegetation to climate in dryland ecosystems: A case study between Xinjiang and Arizona. Remote Sensing, 12(21), 3567. (†: equal contribution)
- Wang, C., Wang, Z.-H., & Li, Q. (2020). Emergence of urban clustering among U.S. cities under environmental stressors. Sustainable Cities and Society, 63, 102481.
- Wang, C., Wang, Z.-H., & Sun, L. (2020). Early-warning signals for critical temperature transitions. Geophysical Research Letters, 47(14), e2020GL088503.
- Wang, C., & Wang, Z.-H. (2020). A network-based toolkit for evaluation and intercomparison of weather prediction and climate modeling. Journal of Environmental Management, 268, 110709.
- Wang, C., Wang, Z.-H., Wang, C. Y., & Myint, S. W. (2019). Environmental cooling provided by urban trees under extreme heat and cold waves in U.S. cities. Remote Sensing of Environment, 227, 28–43.
- Wang, C., Wang, C. Y., Myint, S. W., & Wang, Z.-H. (2017). Landscape determinants of spatio-temporal patterns of aerosol optical depth in the two most polluted metropolitans in the United States. Science of the Total Environment, 609, 1556–1565.
- Wang, C., & Wang, Z.-H. (2017). Projecting population growth as a dynamic measure of regional urban warming. Sustainable Cities and Society, 32, 357–365.